By Richard Ratliff, Executive Scientist at Sabre Labs, and Sergey Shebalov, VP and Head of Research at Sabre Labs
In Part 1 of this three-part series looking at advanced AI/ML applications for airlines, we explored the widespread (and growing) use of AI/ML in the airline industry today, along with the first of five new AI/ML applications: dynamic pricing and availability of airfares.
In this second part, we consider three more new AI/ML applications:
Air ancillary dynamic pricing
Experimentation engines
Market size forecasting
Air ancillary dynamic pricing
One of the biggest trends in airline marketing over the past 10 years has been the strong and steady growth of ancillary sales for air travel. According to a 2022 report from IdeaWorks, airline ancillary revenue trends since 2013 show an average estimated annual growth rate of more than 15%. Ancillary sales growth in this period arises from both individual ancillary sales (a la carte) and the proliferation of airline branded fare bundles.
In the past, ancillary prices were a secondary consideration by airline marketing teams, and those prices were usually static (and often relatively constant) across airline regions. But as sales (and revenues) have increasingly shifted to ancillaries, the opportunities for more dynamic pricing with AI/ML technology have grown. AI/ML models can help airlines recommend optimal prices for ancillary products or services using a mix of supervised learning (based on estimated price elasticities and factors like marketing segment, type of seat, position in cabin, etc.) and reinforcement learning methods, which will be described later in this article. At Sabre, ancillary optimization models are mainly focused on pricing of premium seats and bags, but we are also building extensions to upgrades, other ancillaries and ancillary bundles.

AI/ML models can help airlines recommend optimal prices for ancillary products or services using a mix of supervised learning (based on estimated price elasticities and factors like marketing segment, type of seat, position in cabin, etc.) and reinforcement learning methods, which will be described later in this article. At Sabre, ancillary optimization models are mainly focused on pricing of premium seats and bags, but we are also building extensions to upgrades, other ancillaries and ancillary bundles.
In practice, our customers have seen incremental ancillary revenue gains of up to 10% from the use of AI/ML models for both dynamic price decreases and increases of ancillaries. For more information:
Experimentation engines
One of the applications we’ve developed in Sabre Labs that we’re most excited about is our new experimentation engine platform, based on a type of AI/ML known as reinforcement learning.
How is the experimentation engine different from traditional AI/ML methods?
Traditional AI/ML models analyze a lot of historical data and build complex prediction models relying on historical data. Experimentation engines, however, do not rely on extensive historical data; they just try out (i.e. actively experiment) multiple options and observe the outcomes. The models learn from those outcomes, so the parameters that produce outputs are adjusted a bit, and the process can be run again (and again, and again). After numerous tries, the experimentation engine continues to refine the underlying statistical distributions of the outcomes and can automatically learn which approaches perform best under different circumstances.
One example use case is Sabre Hospitality’s SynXis® Retail Studio™ application for hotels, which has many similarities to airline retailing applications. A hotel can have many different ancillary products and choosing the right ones to present to each guest type is a complicated problem. It’s a huge advantage having access to a self-learning AI/ML model that, in conjunction with the hoteliers’ retail strategies, can try out different ancillary offers to different guest types and (over time) automatically learn which ones work best for each guest type.
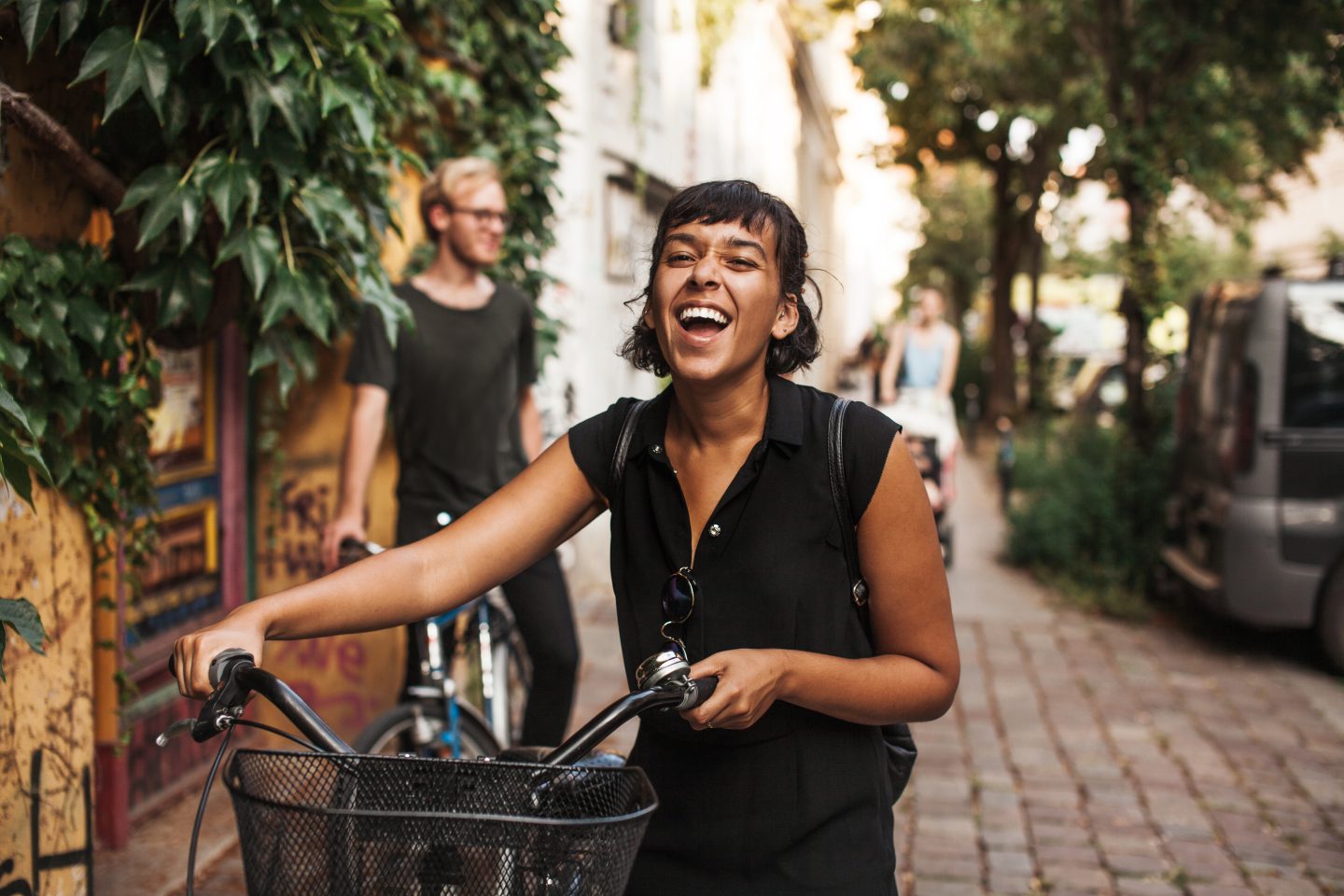
The two graphs below demonstrate how the experimentation engine model learns which product is the best one to offer to two different customer segments, A and B. At first (see the left side of the graphs), the model offers all three products. With time, it settles on a clear solution: A prefers breakfast and B prefers high floor. The model learns as it gets more data points (from impressions and clicks), so the degree of ’exploration’ can be reduced as it gains confidence about the best products to display.
Hotel ancillary offer optimization example:
Automatically finding the best customized offer for each customer segment

In practice, our hotel customers have observed up to 30% gains in guest clickthrough rates when using the experimentation engine for ancillary offer display decisions vs. a uniform display strategy.
Market size forecasting
Two of the most important questions in airline network planning when designing future schedules are understanding “Where do people want to go?” and “How many people want to travel between those origin-destination pairs?”. If we know both answers, we know travel demand, and hence we can help optimize travel supply (i.e. determining the number of planes needed, where they should fly to and how they should be scheduled). That means we can build systems that estimate expected revenue and profitability, fine tune retailing and distribution strategies, plan resources, etc.
Accurately answering “How many people want to travel from city A to city B?” is important for aircraft manufacturers, airlines, hotel operators, travel agencies, local businesses oriented to tourism and the investment community. There are about 250,000 city pairs in the world that have air service between them, and travel demand for them is changing every month; thus, answering that question with manual market analysis is virtually impossible.
This is where Sabre’s new network planning tools can help. Our Sabre Labs team is working on an AI/ML model known as the “Market Potential Forecaster” which predicts how many people would travel from A to B per month for the next 12 months for all 250,000 markets. And it updates such predictions periodically. The underlying AI/ML model is trained on both historical trends and forward-looking air low fare search shopping data. Our colleague, Cuneyd Kaya, provided further model details in this recent Sabre article.
By using a combination of historical data, analyzing segment behaviors and forward-looking shopping data, the AI/ML-based market potential forecaster model has the potential to increase average prediction accuracy to >90% for customers using our solutions.
Read Part 3 and final installment of this three-part series in which we examine new tools for handling disrupted passenger re-accommodation as well as explaining how cloud-based computing and improved machine learning operations (MLOps) are making AI/ML more useful and powerful than ever.
About the Authors

Richard Ratliff, Executive Scientist at Sabre Labs, has held roles of increasing responsibility in IT at Sabre for over 30 years with a focus on travel retailing and revenue management.

Sergey Shebalov, VP and Head of Research at Sabre, leads a team responsible for development and implementation of decision support systems in the travel industry. Sergey holds PhD in math from University of Illinois and has two decades of experience in airline and agency IT.
Keep the lines of communication open
Be the first to know when we release new information on emerging technology, upgrading of existing systems, or updates to our point of view on the future of travel retail.