At the AGIFORS (Airline Group of the International Federation of Operational Research Societies) meeting that I recently attended in Toulouse, revenue management executives at two major airlines asked me: “What is the best way to segment my customers for marketing purposes?” It’s a question that doesn’t necessarily have one right answer, but I thought it was an interesting topic worthy of discussion.
I think it’s helpful to distinguish three different types of customer segmentation, each of which are useful for different marketing purposes, and may in fact be considered simultaneously. Think of them as three different “lenses” through which the customer can be viewed.
- Anonymous, broad-scale segmentation classifications that are useful for describing major types of customers (on an aggregate basis) which are universally applicable across a wide range of use cases. Anonymous segmentation schemes do not rely on individually identifiable information and are thus respectful of data privacy considerations. They are also useful in situations where the customer is not uniquely known and help to provide shopping results and/or offers that can be targeted to the aggregate segment.
- Customer-specific segmentation for situations in which the actual customer details are known. The classic RFM (recency, frequency, monetary) dimensions are often applied in this segmentation scheme from the customer’s past purchase behavior. It may also include other considerations such as declared customer preferences, loyalty program affiliation, demographics, airline website activity, company affiliations, preferred agencies, etc., all of which can help provide more relevant personalized offers.
- Application or use-case specific segmentation of customers. In many industries, the term “cohort” is used to describe a group of individuals with a common set of characteristics, and in this article, we’ll use the term cohort to describe a use-case specific segmentation classification (e.g., cohorts used for the “spring break promotion” or for “in-flight sales”), so the meaning of cohorts is flexible and unique to a given use case.
Anonymous, broad-scale segmentation
Sabre’s “trip-purpose segmentation” is an example of a general segmentation classification scheme that is broadly applicable across a range of uses and analyses. Trip-purpose segmentation is based on characteristics such as advance purchase, length of stay, number in party, number of children, one-way or roundtrip air, country point-of-sale, type of agency (e.g., OTA, corporate, etc.), language, or similar factors associated with the trip request. Personalization via “anonymous segmentation” is very important in travel marketing because oftentimes the customer is not specifically known; even when a customer is uniquely identifiable, historical data pertaining to that customer may be sparse (i.e., approximately 70% of customer travel with an airline no more than once a year). So, trip-purpose type is valuable as a universally applicable mechanism for personalization.
What constitutes a “good” trip-purpose segmentation classification? First you must determine the best number of clusters and that varies from organization to organization. Fewer defined segments make it easier to understand the classification scheme, whereas more segments allow for greater target specificity in marketing. At most companies, the number of customer segments typically ranges from 5 – 25, but some companies have reported using hundreds or even thousands of different segments. Obviously, the higher the number of customer segments, the more difficult it is for marketing analysts and managers to track and manage, so beyond a certain point, adding more segments requires advanced machine learning tools and less reliance on users.
To maximize clarity and minimize overlap in the segmentation definitions, it is recommended that the segmentation classifications have the MECE property (mutually exclusive and collectively exhaustive). Being MECE implies that the definitions cover 100% of all possible trip requests, and any one particular request will fall into one – and only one – trip-purpose segment. When classified in this manner, trip-purpose segmentation becomes an easily understandable “glass box” (rather than an obtuse “black box”).
Furthermore, a good segmentation classification scheme will spread demand across the segments (not necessarily evenly though); if a particular segment has only a relatively small amount of demand associated with it, the airline may consider combining it with another segment for simplicity. Segments are considered well separated when they exhibit minimum variation of requests mapped within a given segment and maximum variation across the different segments. Although it is common for airline segmentation definitions to be manually defined, machine learning tools for unsupervised learning (like clustering models), that minimize intra-cluster distance metrics and maximize inter-cluster ones, are also often used for this purpose.
Finally, a good segmentation scheme will exhibit variety in the buying and usage patterns across different segments. The example below shows the standard trip-purpose segmentation scheme currently in use at Sabre Labs. Published research reports by Sabre demonstrate that customer choice models fitted to our standard default trip-purpose segments exhibit distinct variation on price versus schedule sensitivity by segment, and they also show strong differences in ancillary preferences by segment. These results imply that there are differences in customer behavior across the segments, and that’s an indication that the segmentation scheme is applicable for targeted marketing. Trip-purpose segmentation is useful during the shopping and sales processes, but it is also valuable for any general marketing analyses.
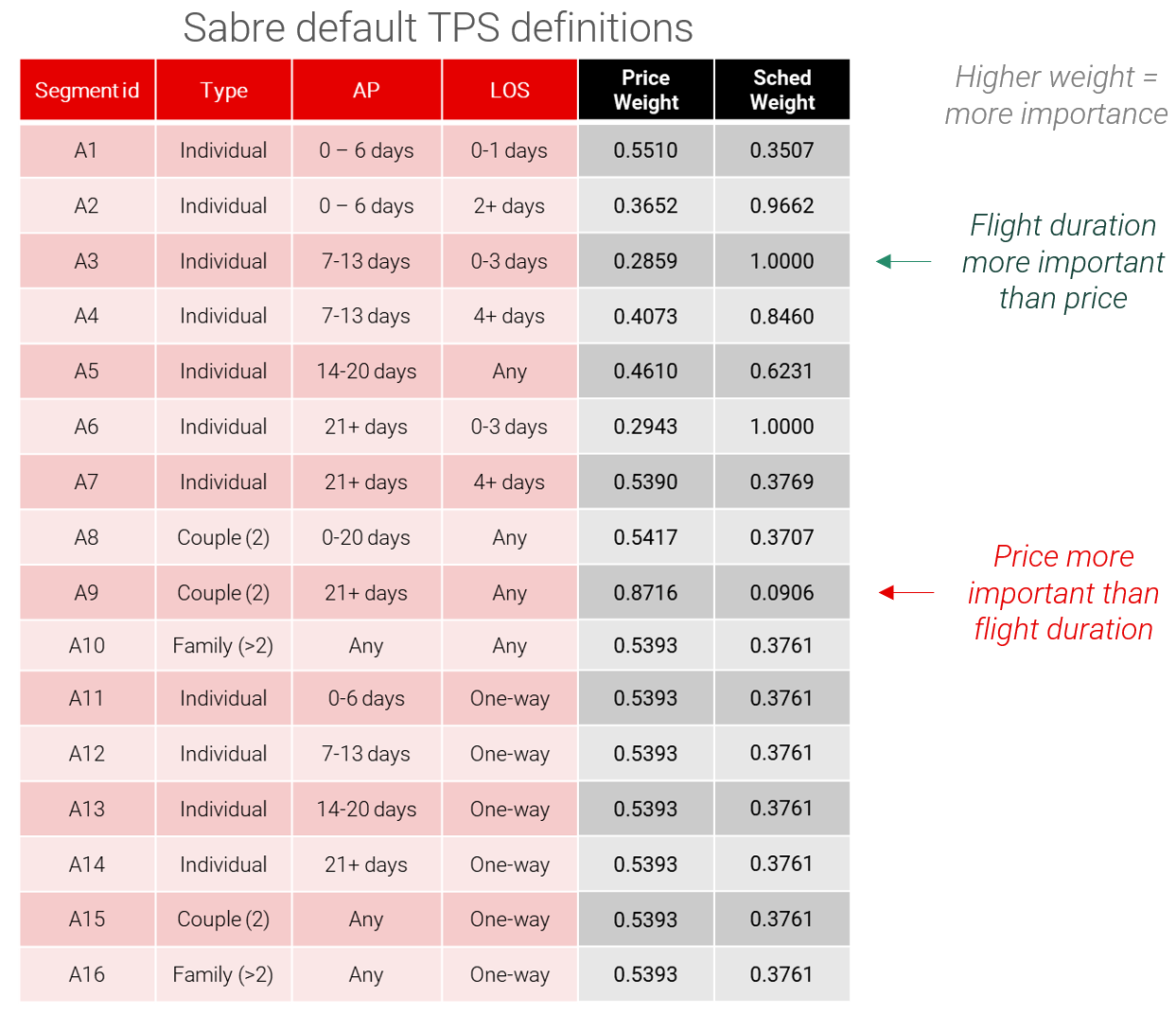
Figure 1: Sabre’s default trip-purpose segmentation classification with indicative price and schedule preferences
Customer-specific segmentation
Most of the classical marketing literature is directed at “known” customers. Air travel purchase and security requirements involve gathering customer identity information, so post-purchase airline marketing situations involve known customers. In some cases, even the shopping and planning phases can involve known customers, e.g., when customers log in to the airline websites, have tracking cookies enabled, or are using a travel agent who provides traveler details for special marketing incentives (e.g., frequent flyer benefits, corporate discounts, etc.).
A widely used approach for managing customer-specific details is the recency, frequency, monetary (RFM) spend model. “Recency” information is straightforward – it’s how long it has been since the customer’s last purchase. It allows airlines to generate different types of offers based on recency information (e.g., “It’s been a while since we last served you…” vs. “Here’s another destination you might be interested in based on your recent trip with us…”). “Frequency” information is chronological data on the customer’s prior purchases and includes statistics such as average days between purchases, average number of items per purchase, etc. “Monetary” information is associated with the historical revenue spend; it can be the total revenue across the historical period considered, average revenue per purchase, weighted average revenue (skewed towards more recent transactions) or other similar metrics. RFM data can be used to determine both what and when things were purchased by the customer – obviously useful in generating relevant personalized offers. Taken together, the three types of information in the RFM model provide a helpful way to organize historical transactional information.
This customer-specific information has the potential to enable the airline to generate truly personalized offers that are customized to the individual’s preferences and needs. Google is currently working with Sabre on an initial prototype to help deliver such personalized offer generation capabilities using a new “Travel Data Platform” (TDP). Once ready, it is expected to allow for persona-based marketing that is tailored to include relevant history and special circumstances in generating the offer; e.g., provide access to additional complementary features due to a recent operational disruption that the traveler faced or a new hotel recommendation based on a newly added leisure activity. So stay tuned for more news about this project.
Application or use-case specific segmentation
The third category is app-specific customer segments; these classification schemes can be thought of as “temporary” or “additional” cohorts that are meaningful to a particular marketing promotion, application, or analysis. To avoid confusion, in this article, we use the term “cohort” in the context of app-specific segmentation. Thus, we can describe a unique “cohort” for a particular marketing application which can include either or both anonymous and/or customer-specific aspects (e.g., late-booking, single traveler, marquis-member frequent flyers who have traveled in the past year and work for Google are being considered for a new marketing promotion in the San Francisco Bay area). Such cohort definitions are very flexible and can be quite specific for a given promotion; furthermore, they often involve using a combination of trip-purpose and customer-specific information.
Conclusion
All three of the different views of customer segmentation views are applicable in practice. Their usage varies depending on the:
- Context (in terms of the available information at the time of the customer contact), and the
- Specific marketing function under consideration
In summary, each of these views are simply different “lenses” through which to view the customer, and in practice all three of them may be considered together when conducting personalized marketing activities.
Want to learn more? See: https://www.sabre.com/retail-intelligence/